As AI applications continue to grow in complexity and scale, engineering teams are looking for better ways to manage distributed compute. Tools like Ray and Ray Serve have become popular choices for teams building scalable inference and training pipelines—but deploying and managing them on Kubernetes introduces operational overhead most teams would rather avoid. That’s where KubeRay comes in.
KubeRay is the Kubernetes-native way to run Ray clusters. And when paired with Kapstan, managing Ray workloads becomes as easy as flipping a switch. Whether you’re scaling model inference with Ray Serve or orchestrating parallel compute jobs with Ray itself, Kapstan eliminates the Kubernetes complexity—so your team can focus on ML outcomes, not YAML files.
What is the "Ray" of "KubeRay", and Why It Matters:
Ray is a distributed execution framework purpose-built for AI and machine learning workloads. Unlike traditional batch schedulers or job orchestrators, Ray provides native support for:
• Distributed Python and complex workflows
• Hyperparameter tuning and parallel model training
• Serving ML models with autoscaling
• APIs: Simplify creating and scaling APIs that use multiple models
• GPU orchestration for AI acceleration
And thanks to Ray Serve, Ray also provides a robust API for deploying and managing model inference services. This allows engineers to go from training to serving in a single stack.
But to take full advantage of Ray, you need infrastructure that can scale with it. That’s where Kubernetes and KubeRay come in.
What Is KubeRay?
KubeRay is an open-source operator that lets you run Ray workloads on Kubernetes clusters. It abstracts the complexity of setting up Ray head nodes, workers, autoscaling logic, and networking.
With KubeRay, you can:
• Deploy Ray clusters declaratively via Kubernetes manifests
• Leverage Kubernetes-native tools for monitoring, security, and scheduling
• Automatically scale Ray workers based on job demand
KubeRay is powerful—but it’s also complex. Managing headless services, persistent storage, and autoscaling policies can quickly turn into a YAML maze. That’s why Kapstan is the perfect companion.
How Kapstan Helps with KubeRay
Kapstan is a Kubernetes platform built for developers. It brings a GitOps-powered UI to Kubernetes, letting you manage applications and infrastructure without touching Helm charts or raw manifests. When it comes to KubeRay, Kapstan adds value in several ways:
1. One-Click Deployment of KubeRay Clusters
Instead of writing and maintaining KubeRay manifests you can focus on your application logic and let Kapstan ensure it is run on a production grade Ray Cluster. Define your node count, autoscaling rules, and GPU needs—and Kapstan provisions the infrastructure automatically.
2. Autoscaling with Spot Instance Support
Ray workloads often involve bursty, high-volume compute. Kapstan makes it easy to run Ray workers on spot instances, driving down costs while maintaining performance. Built-in automation handles node group creation, taints, and tolerations—no manual tuning required.
3. Simplified Ray Serve Configuration
Need to deploy inference endpoints with Ray Serve? Kapstan integrates easily with Ray Serve’s deployment structure, letting teams configure routes, scaling limits, and model versions via Kapstan’s interface. No need to manage multiple YAML files for each model.
➡️ Learn more: Deploying AI Applications with Ray Serve on Kubernetes (Kapstan Blog)
4. Secrets & Environment Variables Managed Securely
Ray clusters often need access to cloud storage keys, model registries, or custom APIs. Kapstan lets you inject secrets and environment variables into Ray Serve deployments without exposing them in plaintext. All secrets are synced from cloud secret managers like AWS Secrets Manager or HashiCorp Vault.
5. Integrated Monitoring & Rollbacks
Kapstan offers built-in observability for all workloads—including Ray Serve endpoints. Monitor traffic, request times, and resource usage without installing third-party tools. If something breaks, rollback to a previous Ray cluster config or model version with a single click.
Why Kapstan + KubeRay Is the Right Stack
Running Ray on Kubernetes unlocks scalable, distributed compute for AI—but getting started can be overwhelming. Kapstan solves that by providing:
• A developer-friendly UI with GitOps under the hood
• Secure, repeatable deployments of Ray Serve and KubeRay
• Cost-optimized infrastructure with spot instance support
• Simplified secrets, scaling, and monitoring
Whether you’re a startup building your first ML inference pipeline or a data platform team running 100+ Ray workers, Kapstan lets you scale without friction.
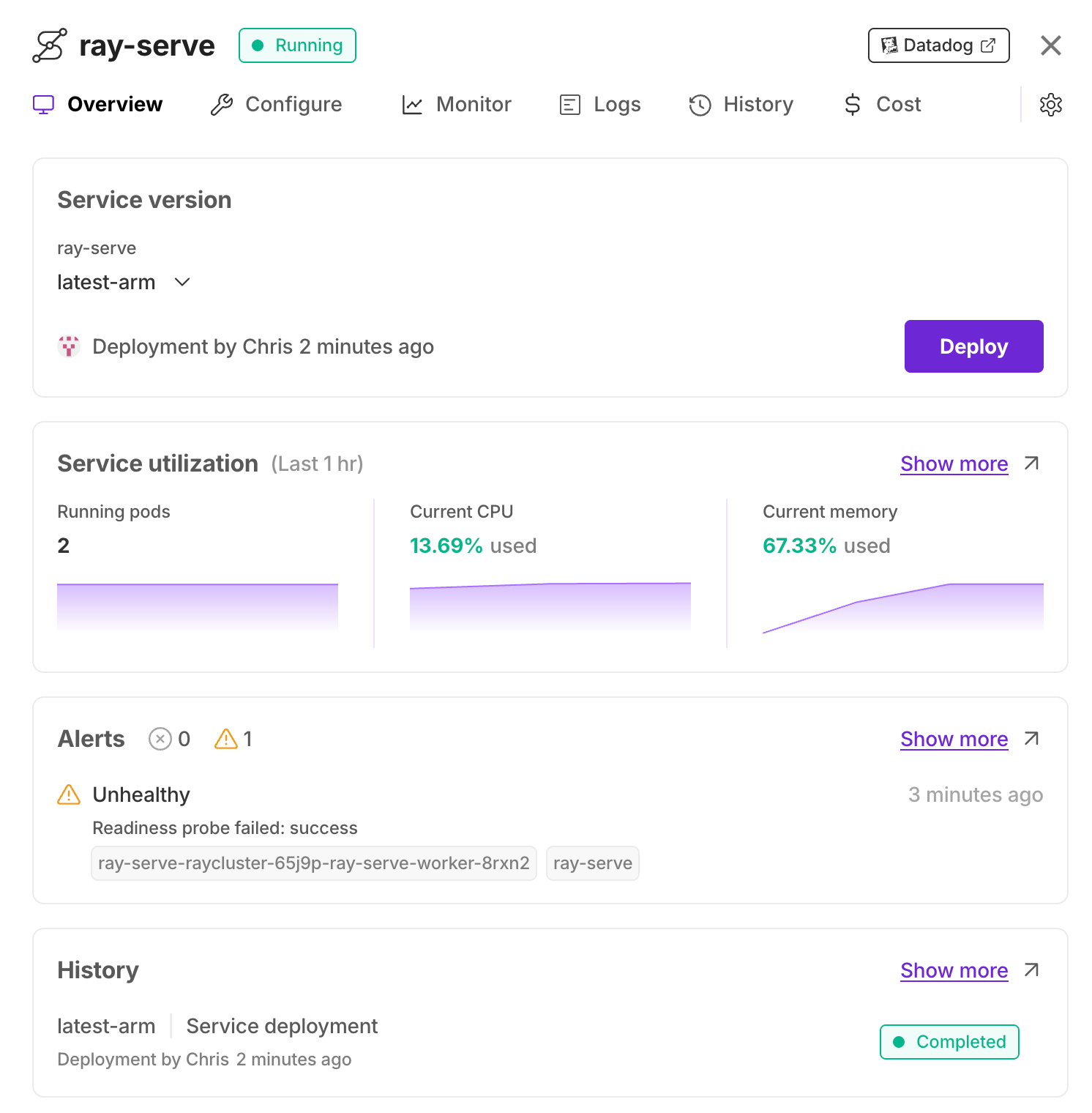
Kuberay: Final Thoughts
AI infrastructure should be powerful—but not painful. KubeRay is a great way to run Ray on Kubernetes, but it still requires heavy DevOps lifting. Kapstan brings simplicity, security, and scalability to KubeRay so teams can move faster with less risk.
If you’re exploring Ray Serve / Kuberay, or building distributed ML services, it’s time to give Kapstan a try.
____________________________________________________________________________________
KubeRay FAQ: Everything You Need to Know
1. What is KubeRay and why is it important for AI workloads?
KubeRay is an open-source Kubernetes operator that simplifies deploying and managing Ray clusters on Kubernetes. It abstracts the complexities of Ray cluster orchestration—including head node management, autoscaling, and networking—making it easier for teams to build scalable machine learning and distributed computing pipelines.
KubeRay is especially useful for AI workloads because it enables:
• Parallel training with Ray
• Inference serving with Ray Serve
• Integration with Kubernetes-native tools for monitoring, autoscaling, and security
2. How does Kapstan simplify working with KubeRay?
Kapstan automates the infrastructure and configuration behind KubeRay so developers don’t have to touch YAML files or manage Helm charts. With Kapstan, teams get:
• One-click deployment of KubeRay clusters
• Seamless Ray Serve configuration
• Spot instance support for cost savings
• Secure secrets injection from cloud providers
• Built-in observability and rollback tools
Learn more: Deploying AI Applications with Ray Serve on Kubernetes (Kapstan Blog)
3. Is KubeRay suitable for production workloads?
Yes, but with caveats. KubeRay is production-ready when properly configured—however, managing it manually can be risky. You’ll need to set up node groups, autoscaling, networking policies, and secure secrets handling. This is where Kapstan adds value—by managing these production-grade configurations automatically and securely.
4. What’s the difference between Ray and KubeRay?
Ray is the distributed compute engine itself, enabling Python-based parallelism, model training, and inference. KubeRay is the Kubernetes-native operator that allows you to run and manage Ray workloads declaratively on Kubernetes.
In short:
Ray = computation layer
KubeRay = infrastructure orchestration layer on Kubernetes
5. Can Kapstan help scale Ray Serve with spot instances?
Absolutely. Kapstan natively supports AWS/GCP spot instances and automatically configures your Ray workers to run on cost-optimized infrastructure. This helps you scale AI workloads without overspending—and without having to configure taints, tolerations, or node affinity manually.
Let me know if you’d like this turned into an HTML version for pasting into Webflow or a CMS.